Data Quality and Technical Notes
Introduction
The estimates produced by the survey are based on information obtained from a sample of persons. Any data collection may encounter factors, known as non-sampling error, which can impact on the reliability of the resulting statistics. In addition, the reliability of estimates based on sample surveys are also subject to sampling variability. That is, the estimates may differ from those that would have been produced had all persons in the population been included in the survey. This is known as sampling error.
Sampling Error
Since the estimates are based on information obtained from a sample of the population, they are subject to sampling error (or sampling variability). That is, the estimates may differ from those that would have been produced had all persons in the population of interest been included in the survey.
The magnitude of the sampling error associated with a survey estimate depends on the following factors:
- Sample design – there are many different methods which could have been used to obtain a sample from which to collect data about experiences of violence. The final design attempted to make key survey results as representative as possible within cost and operational constraints (for further details see the Sample design and selection section of the Sampling chapter of this publication).
- Sample size – the larger the sample on which an estimate is based, the smaller the associated sampling error.
- Population variability – refers to the extent to which people in the target population differ on the particular characteristic being measured. This is referred to as the population variability for that characteristic. The smaller the population variability of a particular characteristic, the more likely it is that the population will be well represented by the sample, and therefore the smaller the sampling error. Conversely, the more variable the characteristic is in the population, the greater the sampling error.
Measures of sampling variability
Sampling error is a measure of the expected difference between published estimates (derived from a sample of persons), and the value that would have been produced if the total population (as defined for the scope of the survey) had been included in the survey. Sampling error is the result of random variation and can be estimated using measures of variance in the data.
One measure of sampling error is the standard error (SE) of the estimate, which indicates the extent to which an estimate might have varied from the true population value due to only a sample of persons being included in the survey. There are about two chances in three (67%) that the sample estimate will differ by less than one SE from the figure that would have been obtained if all persons had been surveyed, and about 19 chances in 20 that the difference will be less than two SEs.
Confidence intervals for example estimate
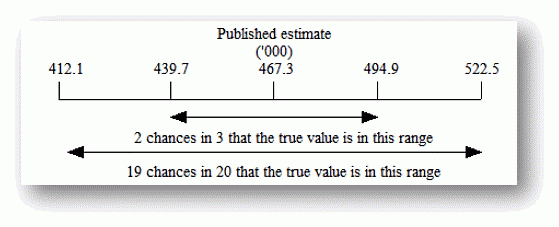
Image displays confidence intervals for an estimate within which a true population value could fall.
For estimates of population sizes, the size of the SE generally increases with the size of the estimate, so that the larger the estimate the larger the SE. However, the larger the survey estimate the smaller the SE becomes in percentage terms. Thus, larger survey estimates will be relatively more reliable than smaller estimates.
Another measure of the likely difference between the survey estimate and the true population value is the relative standard error (RSE), which is obtained by expressing the SE as a percentage of the estimate to which it is related. The RSE is a useful measure as it contextualizes the SE in relation to the size of the estimate.
\(\text{RSE %} = (\frac {SE} { estimate}) \times 100\)
From the 2012 Personal Safety Survey onwards, relative standard errors for estimates are published in 'direct' form. RSEs for estimates are calculated for each separate estimate and published individually using a replicate weights technique (Jackknife method). Direct calculation of RSEs can result in larger estimates having larger RSEs than smaller ones, since these larger estimates may have more inherent variability. More information about the replicate weights technique can be found below.
Estimates with an RSE of less than 25% are considered sufficiently reliable for most purposes. However, estimates with an RSE of 25% or more are also published in the results from the survey. Estimates with an RSE greater than 25% but less than or equal to 50% are annotated with an asterisk (*) to indicate they are less reliable and should be used with caution. Estimates with an RSE of greater than 50% are annotated by a double asterisk (**) and are considered too unreliable for most purposes.
The imprecision due to sampling variability, which is measured by the SE, should not be confused with inaccuracies that may occur because of reporting and/or recording errors made by respondents and/or interviewers during the course of the interview, and data coding and processing errors after enumeration is complete. Inaccuracies of this kind are referred to as non-sampling error, and they may occur in any data collection activity, whether it be a full count (census) or a sample survey. Non-sampling error is caused by factors other than those related to sample selection. It is any factor that results in the data values not accurately reflecting the true value of the population. In practice, the potential for non-sampling error can add to the inaccuracy of the estimates caused by sampling variability. It is not possible to quantify non-sampling error in the same way as sampling error, however every effort is made to reduce non-sampling error to a minimum by careful questionnaire design, intensive training and supervision of interviewers, and efficient operating procedures. For more details on non-sampling error, see below.
Calculation of standard error
The standard error of an estimate can be calculated using the estimate (count or proportion) and its corresponding RSE. For example, according to the results from the 2021-22 Personal Safety Survey (PSS), the estimated number of males aged 18 years and over who experienced physical assault in the last 12 months was 359,000. The RSE provided for this estimate is 19.3%. The standard error is calculated by:
\(\text{SE of estimate} = (\frac {RSE} { 100}) \times estimate\)
= (19.3 / 100) multiplied by 359,000
= 69,300 (rounded to the nearest 100)
Relative standard error of proportions
Proportions formed from the ratio of two estimates are also subject to sampling error. The size of the error depends on the accuracy of both the numerator and denominator. For proportions where the denominator is an estimate of the number of persons in a given population (e.g. all men who experienced physical assault by a male in the last 10 years), and the numerator is the number of persons in the denominator population with a particular characteristic (e.g. men who reported the most recent incident of physical assault by a male to police), a formula to approximate the RSE of the proportion is:
\(\text{RSE} (\frac {x} { y})\approx \sqrt{[RSE(x)]^2-[RSE(y)]^2}\)
This formula is only valid when the numerator (x) is a subset of the denominator (y).
The 2021-22 Personal Safety Survey (PSS) data tables provide relative standard error values for all published proportion estimates. If users wish to calculate the RSE of an unpublished proportion formed from the ratio of two published count estimates (and where one population is a subset of the other), the above approximation formula should be used.
Standard error of a difference
The difference between two survey estimates (counts or proportions) is itself an estimate, and therefore also subject to sampling variability. The sampling error of the difference between two estimates depends on their individual SEs and the level of statistical association (correlation) between the estimates. An approximate SE of the difference between two estimates (x-y) may be calculated using the following formula:
\(\text{SE} (x-y)\approx \sqrt{[SE(x)]^2+[SE(y)]^2}\)
This formula is only valid for differences between discrete and uncorrelated characteristics or sub-populations.
The approximate RSE of a difference between two survey estimates can be calculated from the SE using the following formula:
\(\text{RSE}(x-y)\approx\frac {SE (x-y)} { |x-y|}\)
Standard error of a sum
The sum of two survey estimates (counts or proportions) is itself an estimate, and therefore also subject to sampling variability. The sampling error of the sum of two estimates depends on their individual SEs and the level of statistical association (correlation) between the estimates. An approximate SE of the sum of two estimates (x+y) may be calculated using the following formula:
\(\text{SE} (x+y)\approx \sqrt{[SE(x)]^2+[SE(y)]^2}\)
This formula is only valid for sums of discrete and uncorrelated characteristics or sub-populations.
The approximate RSE of the sum of two survey estimates can be calculated from the SE using the following formula:
\(\text{RSE}(x+y)\approx\frac {SE (x+y)} { x+y} \)
Replicate Weights Technique
A class of techniques called 'replication methods' provide a general method of estimating variances for the types of complex sample designs and weighting procedures employed in ABS household surveys.
The basic idea behind the replication approach is to select sub-samples repeatedly from the whole sample, for each of which the statistic of interest is calculated. The variance of the full sample statistic is then estimated using the variability among the replicate statistics calculated from these sub-samples. The sub-samples are called 'replicate groups', and the statistics calculated from these replicates are called 'replicate estimates'.
There are various ways of creating replicate sub-samples from the full sample. The replicate weights produced for the 2021-22 PSS were created under the delete-a-group Jackknife method of replication (described below).
There are numerous advantages to using the replicate weighting approach, including the fact that:
- the same procedure is applicable to most statistics such as means, percentages, ratios, correlations, derived statistics and regression coefficients
- it is not necessary for the analyst to have available detailed survey design information if the replicate weights are included with the data file.
Derivation of replicate weights
Under the delete-a-group Jackknife method of replicate weighting, weights were derived as follows:
- 60 replicate groups were formed, with each group formed to mirror the overall sample. Units from a cluster of dwellings all belong to the same replicate group, and a unit can belong to only one replicate group.
- For each replicate weight, one replicate group was omitted from the weighting and the remaining records were weighted in the same manner as for the full sample.
- The records in the group that was omitted received a weight of zero.
- This process was repeated for each replicate group (i.e. a total of 60 times).
- Ultimately each record had 60 replicate weights attached to it with one of these being the zero weight.
Application of replicate weights
As noted above, replicate weights enable variances of estimates to be calculated relatively simply. They also enable unit record analyses such as chi-square and logistic regression to be conducted, which take into account the sample design.
Replicate weights for any variable of interest can be calculated from the 60 replicate groups, giving 60 replicate estimates. The distribution of this set of replicate estimates, in conjunction with the full sample estimate, is then used to approximate the variance of the full sample.
The formulae for calculating the standard error (SE) and relative standard error (RSE) of an estimate using this method are shown below:
\(\text{SE} (y)= \sqrt{(\frac{59}{60})\sum_{g=1}^{60}(y_g-y)^2}\)
where:
- g = (1, ..., 60) (the number of replicate weights)
- y(g) = estimate from using replicate weighting
- y = estimate from using full person weight.
The RSE(y) = SE(y)/y*100.
This method can also be used when modelling relationships from unit record data, regardless of the modelling technique used. In modelling, the full sample would be used to estimate the parameter being studied (such as a regression coefficient); i.e. the 60 replicate groups would be used to provide 60 replicate estimates of the survey parameter. The variance of the estimate of the parameter from the full sample is then approximated, as above, by the variability of the replicate estimates.
Availability of RSEs calculated using replicate weights
Actual RSEs for estimates (counts and proportions) have been calculated and are available in spreadsheet format (data cubes) under Data downloads found in the Personal Safety, Australia, 2021-22 publication and the associated thematic releases. The RSEs presented in the data cubes were calculated using the replicate weights methodology described above.
Significance testing of differences between survey estimates
When comparing estimates between surveys or between populations within a survey, it is useful to determine whether apparent differences are 'real' differences or simply the product of differences between the survey samples. One way to examine this is to determine whether the difference between the estimates is statistically significant. This is done by calculating the standard error of the difference between two estimates (x – y) and using that to calculate the test statistic using the formula below (the standard error of the difference between two estimates – the denominator in the below formula – can be calculated using the formula provided in 'Standard error of a difference' located above):
\( (\frac {x-y} { SE (x-y)}) \)
If the value of the test statistic is greater than 1.96, there is good evidence, with a 95% level of confidence, of a statistically significant difference between the two populations with respect to the characteristic being compared. Otherwise, it cannot be stated with confidence (at the 95% level of confidence) that there is a real difference between the populations.
Non-Sampling Error
Non-sampling error may occur in any data collection, whether it is based on a sample or a full count such as a census. Non-sampling errors occur when survey processes work less effectively than intended, and can occur at any stage throughout data collection and processing.
Every effort has been made to reduce non-sampling error through careful design and testing of the questionnaire, training of interviewers, and extensive editing and quality control procedures at all stages of data collection and processing.
The major sources of non-sampling error are:
- response errors due to incorrect interpretation or wording of questions
- errors related to recall and memory
- non-response bias due to non-responders in a sample differing from responders with respect to certain characteristics
- data processing errors, such as mistakes in the recording or coding of the data obtained.
These sources of error are discussed in more detail below.
Response errors
Response errors may have arisen from three main sources:
- flaws in questionnaire design and methodology
- flaws in interviewing technique
- inaccurate reporting by the respondent.
Errors may be caused by misleading or ambiguous questions, inadequate or inconsistent definitions of terminology used, or poor overall survey design (for example, context effects where responses to a question are directly influenced by the preceding questions). In order to overcome problems of this kind, individual questions and the questionnaire overall, were thoroughly tested before being finalised for use in the survey, and interviewers appropriately trained (for more details on testing undertaken and interviewer training, see the Survey Development and Data Collection chapter of this publication).
During testing, particular attention was given to the wording of questions and respondent interpretation of them, as well as to the interviewer instructions, to ensure that information collected fitted within the relevant definitions.
While the questionnaire has been improved and streamlined through rigorous testing, the type and amount of data required from the survey resulted in a complex questionnaire. In some cases, such as when a person had experienced incidents of violence by multiple perpetrators, errors may have resulted from the interviewer and/or the respondent's confusion.
In any survey, inaccurate reporting may occur due to respondents misunderstanding the questions or providing false information to protect their own or others’ privacy and personal safety. For example, some people may not have reported violent incidents they experienced, particularly if the perpetrator was somebody close to them, such as a partner or family member. However, conducting the interviews in private with respondents, and the use of Computer-Assisted Self-Interview (CASI) for the voluntary component of the survey, were procedures implemented to make respondents feel safe and comfortable to disclose sensitive information.
In addition, extensive editing and quality control procedures were applied at all stages of data processing. In situations where known inconsistencies remain in the data that are potentially open to misinterpretation, these are identified in the interpretation section of the relevant content topic chapters of this publication.
Errors related to recall
Recall errors may arise in a number of ways. People may forget to report, or may misreport the details of, incidents that occurred in the past. Recall errors are more likely to occur for information collected about incidents that occurred a long time ago.
To minimise the impact of recall error and reduce respondent burden, when collecting information about the characteristics of a person's most recent incident of the 8 types of violence, detailed information about the most recent incident was only collected if the incident occurred in the last 10 years.
Non-response bias
Non-response occurs when people are unable to or decline to participate in a survey, or are unable to be contacted. Non-response can affect the reliability of results by introducing bias. The impact of any bias depends on the rate of non-response and the extent of the difference between the characteristics of those people who responded to the survey and those who did not.
The 2021-22 PSS achieved an overall response rate of 52.2% (fully responding households, after sample and other loss). It is not possible to quantify the nature and extent of the differences in experiences of violence between respondents in the survey and non-respondents. However, under- or over- representation of particular demographic groups in the sample are compensated for in the weighting process, to achieve representativeness at the state/territory (for female sample), section of state (i.e. capital city and balance of state), sex, age group, and marital status levels. Other disparities are not adjusted for.
The following methods were adopted to reduce the level and impact of non-response:
- use of the Computer-Assisted Self-Interview (CASI) for the sensitive topics, or the alternative options of continuing with a face-to-face interview (Computer-Assisted Personal Interview or CAPI) or a telephone interview (Computer-Assisted Telephone Interview or CATI) with the respondent, conducted in a private setting
- the use of interviewers, where available, who could speak languages other than English (where the language spoken was able to be established)
- follow-up of respondents if there was initially no response after initial contact
- weighting to population benchmarks to ensure national representativeness for key populations of interest.
Interviews where the only questions that were not answered allowed 'don't know' or refusal options (such as income, current partner demographics, or abuse before the age of 15) were treated as fully responding for estimation purposes. These responses were coded to ‘Not known’ or ‘Refusal’ categories as applicable. Furthermore, the characteristics of an additional 2,310 respondents who completed only the compulsory component of the survey were able to be analysed for non-response bias. A selection of characteristics for this population are presented in Response Rates section of the Sampling chapter of this publication.
Processing errors
Processing errors may occur at any stage between the initial collection of the data and the final compilation of statistics. These may be caused by a failure of computer editing programs to detect errors in the data, or may occur during the manipulation of raw data to produce the final survey data files (e.g. while deriving new data items from raw survey data, or during the estimation procedures or weighting of the data file).
To minimise the likelihood of these errors occurring, a number of quality control processes were employed, including:
- within the instruments, trigram coders were used to aid the interviewer with the collection of demographic data, such as education level, country of birth and language spoken. This was complemented by manual coding of text fields where interviewers could not find an appropriate response in the coder.
- computer editing. Edits were devised to ensure that logical sequences were followed in the questionnaires, that necessary items were present, and that specific values lay within certain ranges. These edits were designed to detect reporting and recording errors, incorrect relationships between data items, and missing data items. Following the introduction of the Computer-Assisted Self-Interview option, the number of edits was reduced, with only key edits (such as those associated with perpetrator type and where sequencing would be impacted) applied to the instrument. Where there are known inconsistencies in reporting, these are discussed in the relevant survey content topic chapters.
- data file checks. At various stages during processing (such as after computer editing and subsequent amendments, weighting of the file, and derivation of new data items), frequency counts and/or tabulations were obtained from the data file showing the distribution of persons for different characteristics. These were used as checks on the contents of the data file, to identify unusual values which might have significantly affected estimates, and illogical relationships between data items not previously identified by edits. Further checks were conducted to ensure consistency between related data items, and between relevant populations.
- comparison with historical data. Where possible, checks of the data were undertaken to ensure consistency of the survey outputs against results of previous PSS cycles and comparable data available from other sources.
Other factors affecting estimates
In addition to data quality issues, there are a number of both general and topic-specific factors which should be considered when interpreting the results of the survey. The general factors affect all estimates obtained but may affect specific topics to a greater or lesser degree, depending on the nature of the topic and the intended use of the data. This section outlines these general factors. Topic-specific issues pertaining to the interpretation of data from specific survey topics are discussed in the individual survey content topic chapters of this publication.
Collection mode
Almost half of the respondents (46%) completed their survey as a full face-to-face survey (computer-assisted personal interview or CAPI) conducted by an interviewer. Approximately one-fifth (21%) of respondents completed part of their survey as a CAPI and then opted to complete a computer-assisted self-interview (or CASI). The CASI mode allowed respondents to report their information directly into the questionnaire on the interviewer’s laptop without the need to verbalise their experiences to an interviewer.
Telephone interviewing (computer-assisted telephone interview or CATI) was developed as a contingency during initial nationwide lockdowns during the COVID-19 pandemic. Small-scale testing was carried out in two stages in 2021 before being approved for use in the field in early 2022. Supporting telephone enumeration was critical to achieving collection outcomes, while ensuring interviewers and respondents remained safe and abided by public health guidelines. Approximately one-third (34%) of the sample were interviewed by telephone.
A review of prevalence data by sex and mode did not identify any systematic mode impacts, and the results produced were broadly consistent across the three different data collection modes used to enumerate the survey. Any differences that were found between the modes were small, and consistent with the expectation that there will be some natural variability in results across the mode types. All care was taken to preserve comparability with previous PSS results to enable time series comparisons.
Concepts and definitions
The scope of each topic and the concepts and definitions used therein should be considered when interpreting survey results. This information is available for individual survey content topics in this publication.
Reference periods
All results should be considered within the context of the experiential timeframe that apply to the various topics. Reference periods can differ across topics (e.g. since the age of 15 for violence and stalking prevalence and characteristics of partner violence; before the age of 15 for childhood abuse and witnessing parental violence; last 12 months for sexual harassment; last 10 years for characteristics of the most recent incident) and also across questions (e.g. ‘in the last 12 months’ and ‘in the 12 months after the incident’ for experiences of anxiety or fear). These timeframes should be taken into consideration when comparing results from the survey to data from other sources that may use different reference periods.
Classifications and categories
The classifications and categories used in the survey provide an indication of the level of detail available in survey output. However, the ability of respondents to provide the data may limit the amount of detail that can be output. Classifications used in the survey can be found in in the Classifications and Standards chapter of this publication.
Collection period
The 2021-22 PSS was enumerated from 28 March 2021 to 29 May 2022. When considering PSS results over time, or comparing them with data from other sources, users should take into account any differences between collection periods, and the possible effect of those differences on the data.
Confidentiality (incl. perturbation)
The Census and Statistics Act, 1905, provides the authority for the ABS to collect statistical information, and requires that statistical output shall not be published or disseminated in a manner that is likely to enable the identification of a particular person or organisation. This requirement means that the ABS must take care and make assurances that any statistical information about individual respondents cannot be derived from published data.
To minimise the risk of identifying individuals in aggregate statistics, a technique is used to randomly adjust cell values. This technique is called perturbation. Perturbation involves a small random adjustment of the statistics and is considered the most satisfactory technique for avoiding the release of identifiable statistics while maximising the range of information that can be released. These adjustments have a negligible impact on the underlying pattern of the statistics.
After perturbation, a given published cell value will be consistent across all tables. However, adding up cell values to derive a total will not necessarily give the same result as published totals. The following footnote has been included in all data tables, and diagrams and graphs where required: ‘Cells in this table have been randomly adjusted to avoid the release of confidential data. Discrepancies may occur between sums of the component items and totals.’
Perturbation has been applied to published data from the 2016 PSS onwards. Data from previous PSS editions and the 1996 WSS that are presented in the publications produced for the 2021-22 Personal Safety Survey have not been perturbed, but have been confidentialised to prevent the release of identifiable information about a person if required.